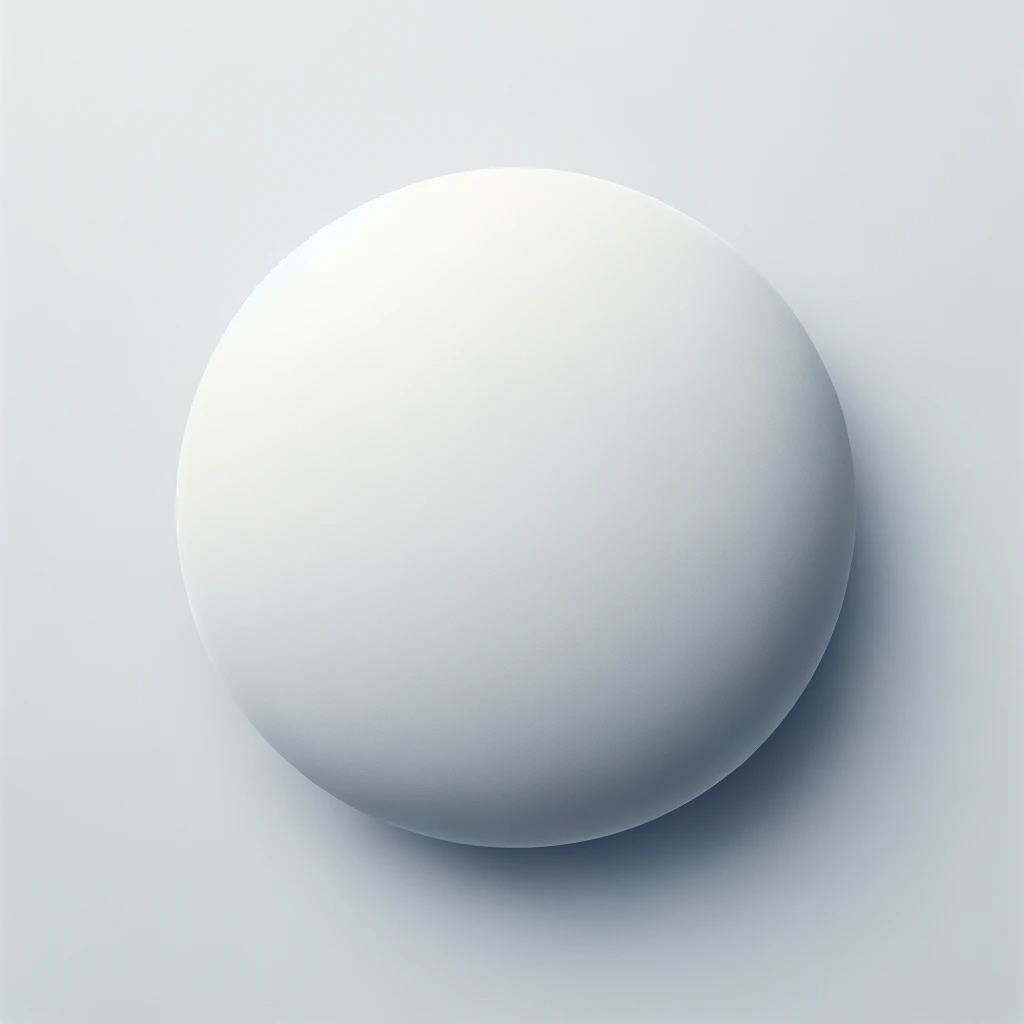
1. ‘Logistic Regression’ is an extremely popular artificial intelligence approach that is used for classification tasks. It is widely adopted in real-life machine learning production settings ...When it comes to traveling with pets, especially when they need to be shipped alone, it’s crucial to find an airline that not only understands the importance of pet safety but also...In today’s fast-paced business world, the success of any company often depends on its ability to effectively manage its supply chain. A key component of this process is implementin...Logistic regression is the appropriate regression analysis to conduct when the dependent variable is dichotomous (binary). Like all regression analyses, the logistic regression is a predictive analysis. Logistic regression is used to describe data and to explain the relationship between one dependent binary variable and one or more nominal, ordinal, …9 Logistic Regression 25b_logistic_regression 27 Training: The big picture 25c_lr_training 56 Training: The details, Testing LIVE 59 Philosophy LIVE 63 Gradient Derivation 25e_derivation. Background 3 25a_background. Lisa Yan, CS109, 2020 1. Weighted sum If !=#!,#",…,##: 4 dot productIntroduction ¶. Logistic regression is a classification algorithm used to assign observations to a discrete set of classes. Unlike linear regression which outputs continuous number values, logistic regression …Small Sample Size: Logistic regression tends to perform better with small sample sizes than decision trees. Decision trees require a large number of observations to create a stable and accurate model, and are more prone to overfitting with small sample sizes. Dealing with Categorical Predictors: Logistic regression can handle categorical ...In deep learning, the last layer of a neural network used for classification can often be interpreted as a logistic regression. In this context, one can see a ...Logistic Regression is a popular statistical model used for binary classification, that is for predictions of the type this or that, yes or no, A or B, etc. Logistic regression can, however, be used for multiclass …Dayton Freight Company is a leading logistics provider that has been in business for over 30 years. They specialize in providing transportation and logistics services to businesses...Configure the Tool · Model name: Each model needs to be given a name so it can later be identified. · Select the target variable: Select the field from the data ...This review introduces logistic regression, which is a method for modelling the dependence of a binary response variable on one or more explanatory variables. Continuous and categorical explanatory variables are considered. Keywords: binomial distribution, Hosmer–Lemeshow test, likelihood, likelihood ratio test, logit function, …According to the University of Connecticut, the criterion variable is the dependent variable, or Y hat, in a regression analysis. The criterion variable is the variable that the an...Then we moved on to the implementation of a Logistic Regression model in Python. We learned key steps in Building a Logistic Regression model like Data cleaning, EDA, Feature engineering, feature scaling, handling class imbalance problems, training, prediction, and evaluation of model on the test dataset.In today’s competitive business landscape, efficiency and streamlined operations are key factors that can make or break a small business. One area that often poses challenges for s...Logistic Regression is not merely a statistical tool but a storytelling device that translates numerical tales into binary outcomes. #2. Introduction to Logistic Regression. Imagine you are at a crossroads where each path leads to a distinct outcome, and your choice is binary: yes or no, true or false, A or B.Logistic regression is a method used to analyze data in order to predict discrete outcomes. The data below is a snapshot of passengers that were on the Titanic. The data shows each passenger ...Logistic regression finds the best possible fit between the predictor and target variables to predict the probability of the target variable belonging to a labeled class/category. Linear …Analisis regresi linier. Seperti yang dijelaskan di atas, regresi linier memodelkan hubungan antara variabel dependen dan independen dengan menggunakan kombinasi linier. Persamaan regresi linier adalah. y = β 0X0 + β 1X1 + β 2X2 +… β nXn + ε, di mana β 1 hingga β n dan ε adalah koefisien regresi.Numerical variable: in order to introduce the variable in the model, it must satisfy the linearity hypothesis,6 i.e., for each unit increase in the numerical ...Numerical variable: in order to introduce the variable in the model, it must satisfy the linearity hypothesis,6 i.e., for each unit increase in the numerical ...Feb 15, 2014 · Abstract. Logistic regression is used to obtain odds ratio in the presence of more than one explanatory variable. The procedure is quite similar to multiple linear regression, with the exception that the response variable is binomial. The result is the impact of each variable on the odds ratio of the observed event of interest. Logistic regression is a statistical model that estimates the probability of a binary event occurring, such as yes/no or true/false, based on a given dataset of independent variables. Logistic regression uses an equation as its representation, very much like linear regression. In fact, logistic regression isn’t much different from linear ...Logistic regression is a method for fitting a regression curve, y = f (x), when y is a categorical variable. The typical use of this model is predicting y given a set of predictors x. The predictors can be continuous, categorical or a mix of both. The categorical variable y, in general, can assume different values.Logistic regression is a very popular type of multiple linear regression that can handle outcomes that are yes versus no rather than numerical values. For example, a regular multiple regression model might deal with age at death as an outcome—possible values being death at age 50, 63, 71, and so forth.In this tutorial, we’ll help you understand the logistic regression algorithm in machine learning.. Logistic Regression is a popular algorithm for supervised learning – classification problems. It’s relatively simple and easy to interpret, which makes it one of the first predictive algorithms that a data scientist learns and applies. ...Logistic regression is a statistical analysis method used to predict a data value based on prior observations of a data set. A logistic regression model predicts a dependent data variable by analyzing the relationship between one or more existing independent variables.Oct 19, 2020 · Logistic regression is just adapting linear regression to a special case where you can have only 2 outputs: 0 or 1. And this thing is most commonly applied to classification problems where 0 and 1 represent two different classes and we want to distinguish between them. Linear regression outputs a real number that ranges from -∞ to +∞. After training a model with logistic regression, it can be used to predict an image label (labels 0–9) given an image. Logistic Regression using Python Video The first part of this tutorial post goes over a toy dataset (digits dataset) to show quickly illustrate scikit-learn’s 4 step modeling pattern and show the behavior of the logistic regression …In Logistic Regression, we wish to model a dependent variable (Y) in terms of one or more independent variables (X). It is a method for classification. This algorithm is used for the dependent variable that is Categorical. Y is modeled using a function that gives output between 0 and 1 for all values of X. In Logistic Regression, the Sigmoid ...In multinomial logistic regression you can also consider measures that are similar to R 2 in ordinary least-squares linear regression, which is the proportion of variance that can be explained by the model. In multinomial logistic regression, however, these are pseudo R 2 measures and there is more than one, although none are easily interpretable.A logistic regression will inform the direction, magnitude, and the statistical significance level of this relationship. In a nutshell, the researcher must use ...Logistic regression is a method used to analyze data in order to predict discrete outcomes. The data below is a snapshot of passengers that were on the Titanic. The data shows each passenger ...Jan 30, 2024 · Binary logistic regression being the most common and the easiest one to interpret among the different types of logistic regression, this post will focus only on the binary logistic regression. Other types of regression (multinomial & ordinal logistic regressions, as well as Poisson regressions are left for future posts). Jan 30, 2024 · The logistic regression model transforms the linear regression function continuous value output into categorical value output using a sigmoid function, which maps any real-valued set of independent variables input into a value between 0 and 1. This function is known as the logistic function. Logistic regression is a statistical model that estimates the probability of a binary event occurring, such as yes/no or true/false, based on a given dataset of independent variables. Logistic regression uses an equation as its representation, very much like linear regression. In fact, logistic regression isn’t much different from linear ...In this video, I explain how to conduct a single variable binary logistic regression in SPSS. I walk show you how to conduct the logistic regression, interpr...In Logistic Regression, we maximize log-likelihood instead. The main reason behind this is that SSE is not a convex function hence finding single minima won’t be easy, there could be more than one minima. However, Log-likelihood is a convex function and hence finding optimal parameters is easier.Logistic regression is a statistical technique that allows the prediction of categorical dependent variables on the bases of categorical and/or continuous independent variables (Pallant, 2005; Tabachnick & Fidell, 2007). Logistic regression assumptions relate to sample size, multicollinearity and outliers.And that last equation is that of the common logistic regression. Understanding Third Variables in Categorical Analysis. Before trying to build our model or interpret the meaning of logistic regression parameters, we must first account for extra variables that may influence the way we actually build and analyze our model.Logistic Regression is basic machine learning algorithm which promises better results compared to more complicated ML algorithms. In this article I’m excited to write about its working. Starting offIn today’s fast-paced digital era, the logistics industry plays a crucial role in ensuring the smooth flow of goods from point A to point B. With increasing demands for efficient a...Logistic regression, also known as logit regression or logit model, is a mathematical model used in statistics to estimate (guess) the probability of an event occurring having been given some previous data. Logistic regression works with binary data, where either the event happens (1) or the event does not happen (0).Binary Logistic Regression: In the binary regression analysis model, we define a category by only two cases. Yes/No or Positive/Negative. Multinomial Logistic Regression: Multinominal logistic analysis works with three or more classifications. If we have more than two classified sections to categorize our data, then we can use this …Sep 13, 2000 ... From the reviews of the First Edition. "An interesting, useful, and well-written book on logistic regression models .In this text, author Scott Menard provides coverage of not only the basic logistic regression model but also advanced topics found in no other logistic ...In logistic Regression, we predict the values of categorical variables. In linear regression, we find the best fit line, by which we can easily predict the output. In Logistic Regression, we find the S-curve by which we can classify the samples. Least square estimation method is used for estimation of accuracy.Linear regression and logistic regression are the two widely used models to handle regression and classification problems respectively. Knowing their basic forms associated with Ordinary Least Squares and Maximum Likelihood Estimation would help us understand the fundamentals and explore their variants to address real-world problems, …Dec 31, 2020 ... Logistic regression is a statistical model that in its basic form uses a logistic function to model a binary dependent variable, although many ...Logistic regression is a method used to analyze data in order to predict discrete outcomes. The data below is a snapshot of passengers that were on the Titanic. The data shows each passenger ...In this tutorial, we’ve explored how to perform logistic regression using the StatsModels library in Python. We covered data preparation, feature selection techniques, model fitting, result ...Logistic Regression Overview. Math Prerequisites. Problem Formulation. Methodology. Classification Performance. Single-Variate Logistic Regression. Multi-Variate Logistic …Sep 13, 2000 ... From the reviews of the First Edition. "An interesting, useful, and well-written book on logistic regression models .In this tutorial, we’ll help you understand the logistic regression algorithm in machine learning.. Logistic Regression is a popular algorithm for supervised learning – classification problems. It’s relatively simple and easy to interpret, which makes it one of the first predictive algorithms that a data scientist learns and applies. ...In today’s fast-paced world, logistics operations play a crucial role in the success of businesses across various industries. Effective transportation management is essential for c...Note: For a standard logistic regression you should ignore the and buttons because they are for sequential (hierarchical) logistic regression. The Method: option needs to be kept at the default value, which is .If, for whatever reason, is not selected, you need to change Method: back to .The "Enter" method is the name given by SPSS Statistics to standard …With logistic regression, we are not trying to predict a continuous value, we’re modeling the probability that an input variable belongs to the first/default class. This is where the sigmoid function comes in. Image by author. Where e is Euler’s number and t is the continuous output from the linear function.Whereas logistic regression is used to calculate the probability of an event. For example, classify if tissue is benign or malignant. 11. Linear regression assumes the normal or gaussian distribution of the dependent variable. Logistic regression assumes the binomial distribution of the dependent variable. 12.Logistic regression is a statistical method used to model the probability of a binary outcome given an input variable. (David O. Manz et al., 2017) It is commonly used in fraud detection to identify patterns and relationships between dependent binary variables. (Pritheega Magalingam et al., 2021) Logistic regression works by performing … Logistic regression is a generalized linear model where the outcome is a two-level categorical variable. The outcome, Yi, takes the value 1 (in our application, this represents a spam message) with probability pi and the value 0 with probability 1 − pi. It is the probability pi that we model in relation to the predictor variables. Configure the Tool · Model name: Each model needs to be given a name so it can later be identified. · Select the target variable: Select the field from the data ...Linear regression and logistic regression are the two widely used models to handle regression and classification problems respectively. Knowing their basic forms associated with Ordinary Least Squares and Maximum Likelihood Estimation would help us understand the fundamentals and explore their variants to address real-world problems, …Regression techniques are versatile in their application to medical research because they can measure associations, predict outcomes, and control for confounding variable effects. As one such technique, logistic regression is an efficient and powerful way to analyze the effect of a group of independent vari-ables on a binary outcome by ...Logistic regression enables you to investigate the relationship between a categorical outcome and a set of explanatory variables. The outcome, or response, can be dichotomous (yes, no) or ordinal (low, medium, high). When you have a dichotomous response, you are performing standard logistic regression. When you are modeling an …Oct 11, 2021 · 📍 Logistic regression. Logistic regression is a binary classification algorithm despite the name contains the word ‘regression’. For binary classification, we have two target classes we want to predict. Let’s refer to them as positive (y=1) and negative (y=0) classes. When we combine linear regression and logistic function, we get the ... Principle of the logistic regression. Logistic regression is a frequently used method because it allows to model binomial (typically binary) variables, ...Consequently, Logistic regression is a type of regression where the range of mapping is confined to [0,1], unlike simple linear regression models where the domain and range could take any real …Logistic regression is a method we can use to fit a regression model when the response variable is binary.. Logistic regression uses a method known as maximum likelihood estimation to find an equation of the following form:. log[p(X) / (1-p(X))] = β 0 + β 1 X 1 + β 2 X 2 + … + β p X p. where: X j: The j th predictor variable; β j: The coefficient …Binary Logistic Regression is useful in the analysis of multiple factors influencing a negative/positive outcome, or any other classification where there are only two possible outcomes. Binary Logistic Regression makes use of one or more predictor variables that may be either continuous or categorical to predict the target variable classes.Hop on to module no. 4 of your machine learning journey from scratch, that is Classification. In this video we will discuss all about Logistic Regressions, w...Mar 31, 2023 · Logistic regression is a popular classification algorithm, and the foundation for many advanced machine learning algorithms, including neural networks and support vector machines. It’s widely adapted in healthcare, marketing, finance, and more. In logistic regression, the dependent variable is binary, and the independent variables can be ... Generate Example Data. To illustrate the differences between ML and GLS fitting, generate some example data. Assume that x i is one dimensional and suppose the true function f in the nonlinear logistic regression model is the Michaelis-Menten model parameterized by a 2 × 1 vector β: f ( x i, β) = β 1 x i β 2 + x i.In this doctoral journey (http://thedoctoraljourney.com/) video, Dr. Rockinson-Szapkiw shows you how to conduct a logistic regression using SPSS.Learn what logistic regression is, how it differs from linear regression, and how to use it for binary and multiclass classification problems. See the …Logistic regression is one of the most frequently used machine learning techniques for classification. However, though seemingly simple, understanding the actual mechanics of what is happening — odds ratio, log transformation, the sigmoid — and why these are used can be quite tricky.Logistic regression is an efficient and powerful way to assess independent variable contributions to a binary outcome, but its accuracy depends in large part on careful variable selection with satisfaction of basic assumptions, as well as appropriate choice of model building strategy and validation of results.Logistic Regression Marketing example data Medical example data. Logistic regression is a special case of regression analysis and is used when the dependent variable is nominally scaled.This is the case, for example, with the variable purchase decision with the two values buys a product and does not buy a product.. Logistical regression analysis … 9 Logistic Regression 25b_logistic_regression 27 Training: The big picture 25c_lr_training 56 Training: The details, Testing LIVE 59 Philosophy LIVE After training a model with logistic regression, it can be used to predict an image label (labels 0–9) given an image. Logistic Regression using Python Video The first part of this tutorial post goes over a toy dataset (digits dataset) to show quickly illustrate scikit-learn’s 4 step modeling pattern and show the behavior of the logistic regression …Logistic regression is the go-to linear classification algorithm for two-class problems. It is easy to implement, easy to understand and gets great results on a wide variety of problems, even when the expectations the method …Hop on to module no. 4 of your machine learning journey from scratch, that is Classification. In this video we will discuss all about Logistic Regressions, w...Logistic regression returns an outcome of 0 (Promoted = No) for probabilities less than 0.5. A prediction of 1 (Promoted = Yes) is returned for probabilities greater than or equal to 0.5: Image by author. You can see that as an employee spends more time working in the company, their chances of getting promoted increases.Logistic regression is a method used to analyze data in order to predict discrete outcomes. The data below is a snapshot of passengers that were on the Titanic. The data shows each passenger ...Dayton Freight Company is a leading logistics provider that has been in business for over 30 years. They specialize in providing transportation and logistics services to businesses...First, we need to remember that logistic regression modeled the response variable to log (odds) that Y = 1. It implies the regression coefficients allow the change in log (odds) in the return for a unit change in the predictor variable, holding all other predictor variables constant. Since log (odds) are hard to interpret, we will transform it ...A 14-NN model is a type of “k nearest neighbor” (k-NN) algorithm that is used to estimate or predict the outcome of a mathematical query point based on 14 nearest neighbors. The k-...Learn the basic concepts of logistic regression, a classification algorithm that uses a sigmoid function to map predictions to probabilities. See examples, …. Binary logistic regression is one method frequentllogistic (or logit) transformation, log p 1−p. We can make this Multinomial logistic regression is an extension of logistic regression that adds native support for multi-class classification problems. Logistic regression, by default, is limited to two-class classification problems. Some extensions like one-vs-rest can allow logistic regression to be used for multi-class classification problems, although they … Logistic regression is a predictive mode Victorian gambling watchdog says company has addressed failings identified in royal commission but action will be taken ‘if there is any regression to old Crown’ …When it comes to traveling with pets, especially when they need to be shipped alone, it’s crucial to find an airline that not only understands the importance of pet safety but also... Simulating a Logistic Regression Model. Logistic ...
Continue Reading