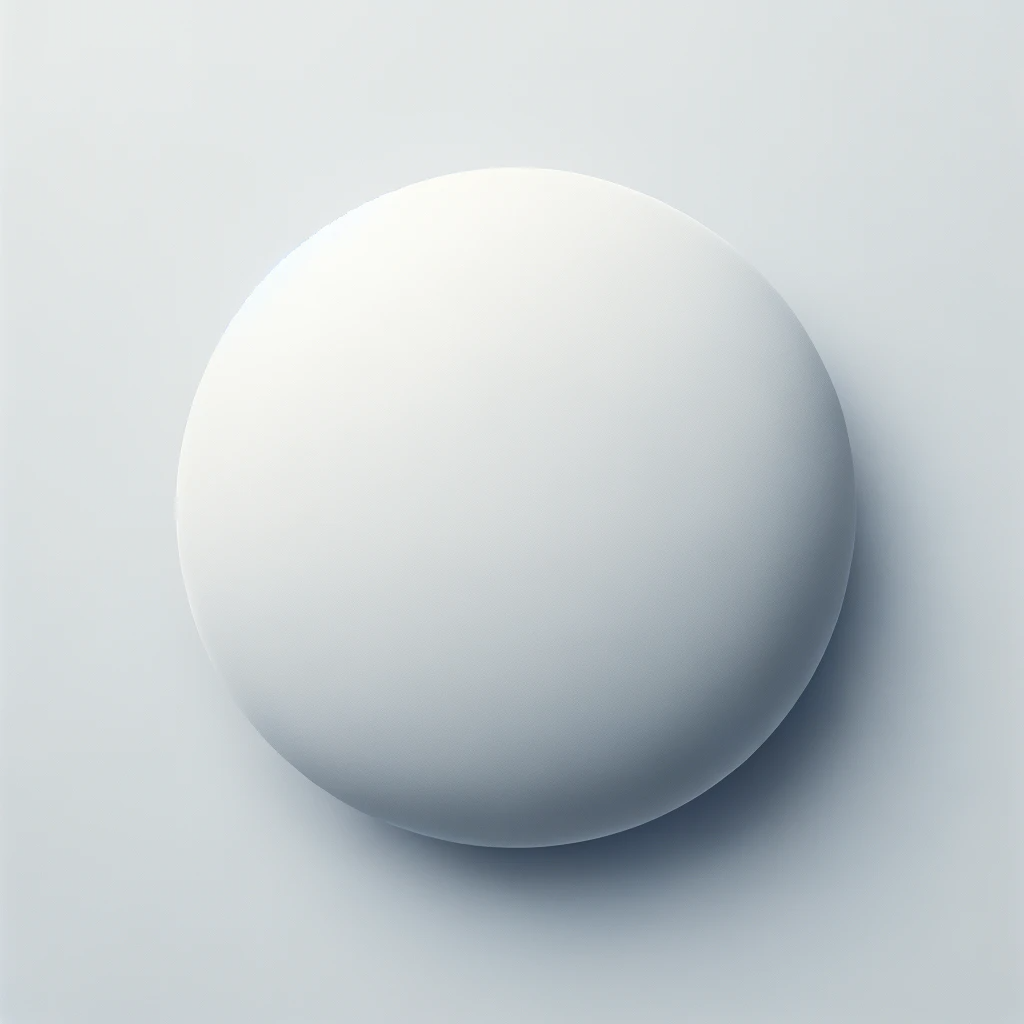
Intro to Generative Adversarial Networks (GANs) by Margaret Maynard-Reid on September 13, 2021. This post covers the intuition of Generative Adversarial Networks (GANs) at a high level, the various GAN variants, and applications for solving real-world problems. This is the first post of a GAN tutorial …A paper that introduces a new framework for estimating generative models via an adversarial process, where a generative model G and a discriminative model D …Learn about the basics, components, and optimization of GANs, a type of neural network that can generate realistic images. See examples of GAN models and …The Super-Resolution Generative Adversarial Network (SR- GAN) is a seminal work that is capable of generating realistic textures during single image super-resolution. However, the hallucinated details are often accompanied with unpleasant artifacts.A generative adversarial network, constrained by the sum of global precipitation, is developed that substantially improves ESM predictions of spatial patterns and intermittency of daily precipitation.Adventure sports clubs and networks let you share your passion for adventure. Visit HowStuffWorks to learn about adventure sports clubs and networks. Advertisement Adventure sports...The generative network keeps producing images that are closer in appearance to the real images while the discriminative network is trying to determine the ...Feb 19, 2021 ... Both the networks will oppose each other to generate synthetic and new data instances, passing the real data. You can use it for video ...Jul 6, 2019 ... The generative model tries to predict features given classes. This involves determining the probability of a feature given a class. It acts as a ...Abstract—Generative adversarial networks (GANs) pro-vide a way to learn deep representations without extensively annotated training data. They achieve this through deriving backpropagation signals through a competitive process in-volving a pair of networks. The representations that can be learned by GANs may be used …The recent advent of generative adversarial networks (GAN) 1,2,3,4,5,6,7 in the creation of high-resolution synthetic images, indistinguishable from real counterparts, has triggered a revolution ...Recent studies have shown remarkable success in image-to-image translation for two domains. However, existing approaches have limited scalability and robustness in handling more than two domains, since different models should be built independently for every pair of image domains. To address this limitation, we …Recently, generative adversarial networks (GANs) have become a research focus of artificial intelligence. Inspired by two-player zero-sum game, GANs comprise a generator and a discriminator, both trained under the adversarial learning idea. The goal of GANs is to estimate the potential distribution of real data samples and …Jul 12, 2019 ... 18 Impressive Applications of Generative Adversarial Networks (GANs) · Generate Examples for Image Datasets · Generate Photographs of Human ...GANs, or Generative Adversarial Networks, are a deep learning mechanism that learns to generate new data samples via a training competition between two models — a generator and …Compared to these methods, our proposed method first exploits the generator network to generate clear and fine faces with high resolution (\(4\times \) up-scaling), and then the discriminator network is trained to distinguish the target faces from the input images.2.3 Generative Adversarial Networks. In the seminal work …Generative Adversarial Networks. Now that we have touched upon other popular generative models, we can take a look at GANs, and how they compare against the rest. GANs were designed to overcome many of the drawbacks stated in the above models. As opposed to Fully Visible Belief Networks, GANs …The generative adversarial network (GAN) framework has emerged as a powerful tool for various image and video synthesis tasks, allowing the synthesis of visual content in an unconditional or input-conditional manner. It has enabled the generation of high-resolution photorealistic images and videos, a task that was challenging or impossible with prior … Generative adversarial networks has been sometimes confused with the related concept of “adversar-ial examples” [28]. Adversarial examples are examples found by using gradient-based optimization directly on the input to a classification network, in order to find examples that are similar to the data yet misclassified. Learn how to create generative models using GANs, a neural network architecture that can generate data similar to humans. Follow a step-by-step tutorial with PyTorch and …In this paper, we propose a multi-scale fusion generative adversarial network named Fusion Water-GAN (FW-GAN) to enhance the underwater image quality. The proposed model has four convolution branches, these branches refine the features of the three prior inputs and encode the original input, then fuse prior …Security and privacy are big concerns these days, particularly when it comes to dealing with sensitive information on the internet. Interested in maintaining your anonymity online?...The purpose of this study is to develop a unified framework for multimodal MR image synthesis. Methods: A unified generative adversarial network consisting of only a single generator and a single discriminator was developed to learn the mappings among images of four different modalities. The generator took an image and its …Two popular approaches are GANs, which are used to generate multimedia, and VAEs, used more for signal analysis. Generative adversarial networks and variational autoencoders are two of the most popular approaches used for producing AI-generated content. In general, GANs tend to be more widely …A generative adversarial network (GAN) has two parts: The generator learns to generate plausible data. The generated instances become negative training …A Generative adversarial network, or GAN, is one of the most powerful machine learning models proposed by Goodfellow et al. (opens in new tab) for learning to generate samples from complicated real-world distributions. GANs have sparked millions of applications, ranging from generating realistic images or …Generative Adversarial Networks. Modeling artificial samples after a given dataset can be done directly by comparing the true data with the generated data, or indirectly by utilizing a downstream task that in turn enables the network to generate real samples. For direct approaches, one can use the Maximum Mean …Apr 1, 2021 · A Generative Adversarial Network (GAN) emanates in the category of Machine Learning (ML) frameworks. These networks have acquired their inspiration from Ian Goodfellow and his colleagues based on noise contrastive estimation and used loss function used in present GAN (Grnarova et al., 2019). Actual working using GAN started in 2017 with human ... Feb 19, 2021 ... Both the networks will oppose each other to generate synthetic and new data instances, passing the real data. You can use it for video ...Jun 10, 2014 · Feb 2024. Generative models are built using an adversarial process that combines two models. A generator that predicts the data distribution and a Discriminator that analyzes the likelihood that a ... This report summarizes the tutorial presented by the author at NIPS 2016 on generative adversarial networks (GANs). The tutorial describes: (1) Why generative modeling is a topic worth studying, (2) how generative models work, and how GANs compare to other generative models, (3) the details of how GANs …Data Augmentation Generative Adversarial Networks. Effective training of neural networks requires much data. In the low-data regime, parameters are underdetermined, and learnt networks generalise poorly. Data Augmentation alleviates this by using existing data more effectively. However standard data …In recent years, the rollout of 5G technology has been making waves across the globe. With its promise of faster speeds, lower latency, and a more connected world, it’s no wonder t...Generative adversarial networks (GANs) have seen remarkable progress in recent years. They are used as generative models for all kinds of data such as text, images, audio, music, videos, and animations. This paper presents a comprehensive review of the novel and emerging GAN-based speech frameworks …Abstract: Generative adversarial Networks (GANs) are a compelling elegance of deep mastering models that can automatically generate synthetic scientific picas from real …Generative adversarial networks (GANs) have seen remarkable progress in recent years. They are used as generative models for all kinds of data such as text, images, audio, music, videos, and animations. This paper presents a comprehensive review of the novel and emerging GAN-based speech frameworks …The purpose of this study is to develop a unified framework for multimodal MR image synthesis. Methods: A unified generative adversarial network consisting of only a single generator and a single discriminator was developed to learn the mappings among images of four different modalities. The generator took an image and its …LinkedIn is not just a platform for professionals to connect with each other; it is also an invaluable tool for companies looking to expand their network, build brand awareness, an...Generative Adversarial Networks (GANs) have been impactful on many problems and applications but suffer from unstable training. The Wasserstein GAN (WGAN) leverages the Wasserstein distance to avoid the caveats in the minmax two-player training of GANs but has other defects such as mode collapse and lack of metric to detect the …Synthesizing high-quality images from text descriptions is a challenging problem in computer vision and has many practical applications. Samples generated by existing text-to-image approaches can roughly reflect the meaning of the given descriptions, but they fail to contain necessary details and …In this paper, we propose the Self-Attention Generative Adversarial Network (SAGAN) which allows attention-driven, long-range dependency modeling for image generation tasks. Traditional convolutional GANs generate high-resolution details as a function of only spatially local points in lower-resolution feature maps. …One of the challenges in the study of generative adversarial networks is the instability of its training. In this paper, we propose a novel weight normalization technique called spectral normalization to stabilize the training of the discriminator. Our new normalization technique is computationally light and easy … The key advantage of generative adversarial networks, or GANs, is that it generates artificial data that is very similar to real data. This is because GANs are made up of 2- neural networks: a generator and a discriminator. As the two networks compete with each other, the generator becomes better at creating realistic data. Discover how to train a generative adversarial network (GAN) to generate image content in DIGITS.Generative adversarial networks consist of two neural networks, the generator, and the discriminator, which compete against each other. The generator is …Introduction. Generative Adversarial Networks takes up a game-theoretic approach, unlike a conventional neural network. The network learns to generate from a …With the rapid advancements in technology, the rollout of 5G networks has become a hot topic of discussion. As more and more devices become compatible with this new generation of w...In a major advance in 2014, Goodfellow et al. 20 introduced generative adversarial networks (GANs). The idea of a GAN is to set up two neural networks, a generative network and a discriminative network, competing with each other. The generator tries to deceive the discriminator by creating fake data samples, whereas the …Recently, there have been claims that generative models such as generative adversarial networks (GANs) can synthesize MR contrasts that are not acquired. However, the poor scalability of existing ...Discover how to train a generative adversarial network (GAN) to generate image content in DIGITS.The adversarial structure can be composed of two competing deep neuron networks, a generative network and a discriminative network. In the GAN-based strategy, we use the discriminative network to map out the relationship between the pixel matrices of elements and their corresponding probability to be …Generative Adversarial Networks (GANs) were introduced in 2014 by Ian J. Goodfellow and co-authors. GANs perform unsupervised learning tasks in machine learning. It consists of 2 models that automatically discover and learn the patterns in input data. The two models are known as Generator and Discriminator.MelGAN: Generative Adversarial Networks for Conditional Waveform Synthesis. Kundan Kumar, Rithesh Kumar, Thibault de Boissiere, Lucas Gestin, Wei Zhen Teoh, Jose Sotelo, Alexandre de Brebisson, Yoshua Bengio, Aaron Courville. Previous works (Donahue et al., 2018a; Engel et al., 2019a) have found that generating coherent … Learn what a generative adversarial network (GAN) is, how it works, and how to train it. A GAN is a deep neural network framework that can generate new data with the same characteristics as a training set. Wasserstein Generative Adversarial Networks. This work introduces a new algorithm named WGAN, an alternative to traditional GAN training that can improve the stability of learning, get rid of problems like mode collapse, and provide meaningful learning curves useful for debugging and hyperparameter searches.Generative adversarial networks (GANs) provide a way to learn deep representations without extensively annotated training data. They achieve this by deriving backpropagation signals through a competitive process involving a pair of networks. The representations that can be learned by GANs may be used in a …Jan 7, 2019 · Learn how GANs work by building the reasoning step by step from the basics of random variable generation. Discover the architecture, the loss function and the examples of GANs, a powerful generative model for machine learning. Generative Adversarial Network framework. GANs are generative models devised by Goodfellow et al. in 2014. In a GAN setup, two differentiable functions, represented by neural …Jul 21, 2022 · By Caper Hansen. Published July 21, 2022. Learn about the different aspects and intricacies of generative adversarial networks (GAN), a type of neural network that is used both in and outside of the artificial intelligence (AI) space. This article walks you through an introduction, describes what GANs are, and explains how you can use them. Wasserstein Generative Adversarial Networks. This work introduces a new algorithm named WGAN, an alternative to traditional GAN training that can improve the stability of learning, get rid of problems like mode collapse, and provide meaningful learning curves useful for debugging and hyperparameter searches.Dec 14, 2022 ... Generative Adversarial Network - the genesis. The idea for Generative Adversarial Network GAN was born in 2014 during a conversation Ian ...Nov 15, 2019 · Recently, generative adversarial networks and in this case specifically cycle consistent generative adversarial networks have enabled a true breakthrough in the quality of synthetic image ... Advertisement The smart grid uses computer technology to improve the communication, automation, and connectivity of the various components of the power network. This allows -- as a...Network security is the combination of policies and procedures implemented by a network administrator to avoid and keep track of unauthorized access, exploitation, modification or ...Oct 25, 2020 ... GANs [1] introduce the concept of adversarial learning, as they lie in the rivalry between two neural networks. These techniques have enabled ...Learn how GANs, a type of neural network, can create new data samples by competing with each other in a bluffing game. Discover different types of GANs, their …Oct 25, 2020 · Generative adversarial networks (GANs) are a generative model with implicit density estimation, part of unsupervised learning and are using two neural networks. Thus, we understand the terms “generative” and “networks” in “generative adversarial networks”. 2.1) The principle: generator vs discriminator The emergence of deep learning model GAN (Generative Adversarial Networks) is an important turning point in generative modeling. GAN is more powerful in ...With the advancement of mobile technology, we are now entering into an era where mobile networks are becoming more advanced and faster. Two of the most popular network technologies...Generative adversarial networks (GANs) are a framework for producing a generative model by way of a two-player minimax game. In this paper, we propose the \\emph{Generative Multi-Adversarial Network} (GMAN), a framework that extends GANs to multiple discriminators. In previous work, the …May 24, 2023 ... Vanilla GANs: Also known as standard GANs, they comprise a generator and a discriminator network. The Generator generates synthetic samples, ...In unsupervised data generation tasks, besides the generation of a sample based on previous observations, one would often like to give hints to the model in order to bias the generation towards desirable metrics. We propose a method that combines Generative Adversarial Networks (GANs) and …May 16, 2020 · Generative Adversarial Networks (GANs) are nothing but a framework for estimating generative models via adversarial process. In this article, we will see, what exactly GANs are, how they work and glance through a few use cases of it. Let’s take a peek into the main contents: Contents. Generative v/s Discriminative Modeling . Discover how to train a generative adversariAffiliate marketing is a popular strategy for anyone looki The Wasserstein Generative Adversarial Network, or Wasserstein GAN, is an extension to the generative adversarial network that both improves the stability when training the model and provides a loss function that correlates with the quality of generated images. It is an important extension to the GAN model and requires a conceptual shift …Within seismology, geology, civil and structural engineering, deep learning (DL), especially via generative adversarial networks (GANs), represents an innovative, engaging, and advantageous way to generate reliable synthetic data that represent actual samples’ characteristics, providing a handy data … Based on recent studies on single image super-reso Generative adversarial networks (GANs), a class of DNNs offer a novel way to model and generate data in an unsupervised manner. The deep learning … Generative Adversarial Networks. Now that w...
Continue Reading